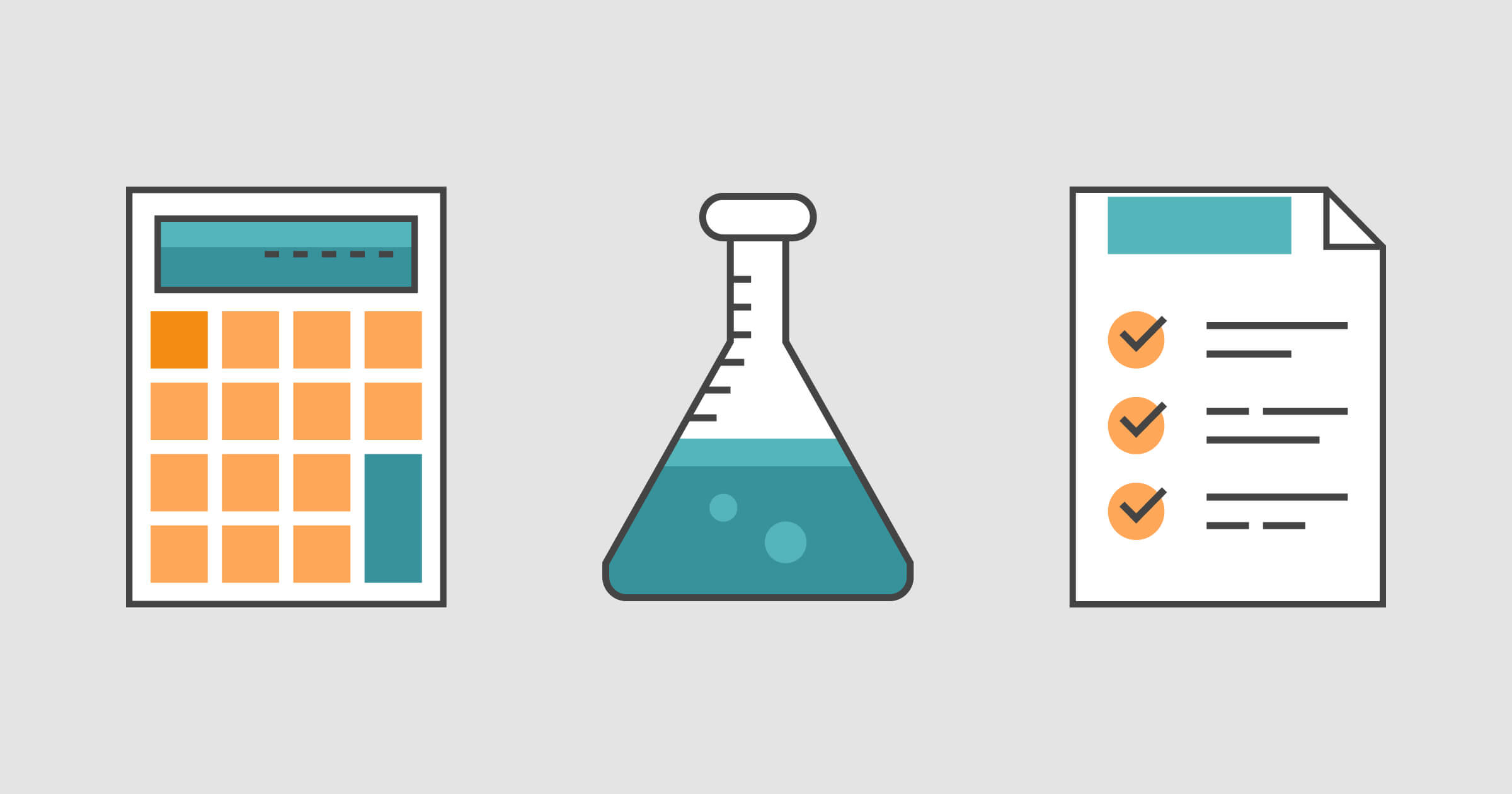
Some Thoughts on Our Cost-effectiveness Estimates
Summary
In this post, we elaborate on our thinking about quantitative cost-effectiveness estimates (CEEs). They’ve been a part of our review process since we began reviewing charities, and they are particularly controversial and challenging. First, we summarize the benefits and challenges of CEEs in effective animal advocacy. Then, we discuss our plan going forward, including the addition of “days of suffering” estimates and trying out the use of ranges instead of point estimates. We especially want to communicate to our audience that CEEs are only one of several perspectives we use in our review process, and that we do not include all available information in our CEEs because some of the subjective or uncertain factors are best accounted for through other perspectives.
A Note Regarding General Uncertainties
We struggle to properly convey the huge amount of uncertainty that goes into our estimates. This is largely because we lack robust evidence, such as high-powered randomized controlled trials, of the effectiveness of animal advocacy interventions. We often have to rely on findings from other fields, case studies of movements and campaigns, and the anecdotal experience of advocates and Researchers. This weakness of evidence makes us more susceptible to biases and other errors. While this uncertainty applies similarly to quantitative and qualitative perspectives, it is more easily noticed in our CEEs, likely because of the concrete numbers involved.
We also want to note that we strongly agree with people who have expressed skepticism about the reliability of our estimates based on the lack of robust evidence.1 We believe that this uncertainty by itself shouldn’t lead to the conclusion that our cost-effectiveness estimates are too high, but it does suggest that the range of plausible estimates is very wide.
Benefits
The primary benefit of CEEs is the potential to compare different interventions in a more objective way than qualitative estimates allow. Let’s take an example of comparing two strategies for hosting a local event to raise awareness for farmed animals: (A) get your friend, who’s not a well-known speaker, to give the keynote speech, (B) reach out to well-known speakers for the keynote speech. You could weigh these options as follows:
Your friend speaks | You try to find a well-known speaker |
It’s guaranteed to work out, but might not bring in as much of a crowd. | They’ll probably turn it down, but if they say yes, attendance will be much higher |
A qualitative evaluation of this situation could be, “It’d be great to have a well-known speaker, but there’s no way they’d say yes, so let’s just go with my friend.” A quantitative evaluation might be, “Hosting a well-known speaker would increase attendance by 500 people and I think it’s 10% likely to be successful. It would only take a few hours of work, and I think the counterfactual use of my time would only increase attendance by 10 people. Based on an expected value of (500 attendees) * (10%) = 50 attendees, it seems like I should really try for the well-known speaker!”
In this example the quantitative estimate had a different outcome and, if you expect your estimates to be reliable, then you’d probably go with that conclusion. Perhaps our qualitative conclusion resulted from not appreciating that even a small chance at a big payoff can be worthwhile, while that factor came through accurately in the quantitative estimate. In general, quantitative estimates seem to work well when we have reliable numbers available and/or when we fear bias in our qualitative analysis.
Challenges
Implementation
When creating CEEs, there are at least two potential difficulties that can make a qualitative analysis more reliable. First is the presence or absence of reliable numbers to use as inputs in a CEE. In the hard sciences, for example, we often have many of these as the results of sophisticated experiments and studies, but we usually lack these when it comes to predicting consumer behavior in response to vegetarian outreach.2
In our work at ACE, we rarely have reliable numbers due to the lack of empirical research, the difficulty charities have with data collection, and the fundamentally subjective nature of some inputs, such as the value of cage-free reforms relative to sparing animals from animal agriculture entirely. We typically have limited information about both the potential value (e.g. number of animals spared from a new corporate welfare policy) and likelihood of success of an intervention.
The second difficulty is the presence of biases that are more prevalent when making the quantitative calculations. For example, the estimates of high-risk, high-benefit programs could be too high. People seem to be more comfortable assigning very large figures to potential benefits than they are assigning very small probabilities to those outcomes, perhaps because we worry too much about overconfidence.3 This difficulty could be less important than the reliability of evidence, but it is exacerbated when we don’t have that reliable evidence to overpower our biases.
Communication
We’ve found CEEs to be the most debated and controversial part of our evaluations, with both our readers and the organizations we review. We think this comes partly from a gut discomfort with assigning numbers to important or hard-to-quantify figures like how much dietary change you expect from receiving a leaflet. If we assign a value of 10% to a figure, some can easily interpret this as our having good reason not to assign 9% or 11%, which implies a level of certainty we rarely achieve.
We’ve heard from some people who interpret our CEEs more literally than we believe they should be, for example, by using our CEE results from top charity reviews to identify one as the most effective.4 We worry about doing this ourselves, especially given that CEE results are a concise way of explaining the huge impact donors can have in animal advocacy.
To help with this issue, we plan on presenting our cost-effectiveness estimates as ranges rather than point estimates in the future. See the next section for details.
Another concern is that because almost any quantitative estimate involves simplification of the factors involved, focusing on CEEs could lead charities or other actors to optimize for measured outcomes in ways that actually decrease overall effectiveness. For example, if we measure social media impact by number of shares, then organizations could overutilize cute videos because these get so many shares, even though the impact-per-share would probably be lower. One way to help would be to make more precise quantitative estimates by accounting for the types of video that an organization shares. We hope to add more precision like this in the future, but we have limited staff time and available information to create these estimates.
Additionally, because we avoid incorporating some of the most uncertain and subjective factors in our quantitative estimates, easy-to-measure outcomes could receive disproportionate consideration. While we still account for the harder-to-measure outcomes in other parts of our reviews, readers could interpret this as the easy-to-measure outcomes being more important.
Our Current Plans
Ranges vs. Point Estimates
We think using ranges, such as 5-20%, will better express our uncertainty than point estimates, such as 12.5%. We think it will emphasize the most useful conclusions from CEEs, when one result is substantially higher than another. When using ranges, we think that if the pessimistic version of one estimate is higher than the optimistic version of another estimate, it’s likely significant evidence that the actual value for the former is higher than the latter. We have not tried this approach yet for charity evaluations, even internally, and we’re unsure of how much overlap the intervals will have because this largely depends on how widely we set them.
We considered a quantitative approach for setting the interval width, such as estimating a 90%, 95%, or 99% prediction interval (such that we think the true value is that likely to lie in the interval). We worry that we don’t usually have enough information to produce prediction intervals from robust statistical analysis, and because using them would give that impression, it might appear overconfident. Instead, we’re leaning towards setting the estimates based on the question, “If we found out the true value of this figure, how low (high) would it have to be to surprise us?” We don’t think this is a great solution, but it currently seems like the best approach we have.5
Finally, even though ranges do a better job of expressing uncertainty, creating them using linear chains of reasoning without sandboxing still leaves them vulnerable to extreme errors at one step of the calculation, while qualitative reasoning tends to limit the influence of those individual factors and keep large errors from making as big of a difference in the final result.
Days of Suffering vs. Lives Spared
In order to make comparisons between interventions that reduce animal suffering in very different ways, such as the difference between one that creates more vegetarians and another that improves the living conditions of farmed animals, we need a common metric. Until now, we’ve used “equivalent number of animals spared from life in industrial agriculture.” This metric emphasizes the number of individuals affected.
We’ve been considering a new metric: “equivalent days of industrial agriculture suffering spared.” This metric aligns more closely with the values many in our audience hold, that we should intrinsically care about the amount of suffering reduced rather than the number of individuals affected.
This would make the biggest difference in our estimates of the effectiveness of humane reforms, where animals are not spared entirely from the cruelty of animal agriculture, as in most interventions we’ve considered, but instead have that suffering reduced, such as by living in an open shed instead of a battery cage. In 2015, we used conversion rates to compare the value of helping an individual in this way to sparing an animal from animal agriculture entirely. These conversion rates didn’t account for the varying lifespans of different farmed animals, such as between a chicken raised for meat (about 1.5 months) and a chicken raised for eggs (about 15.5 months).
Our current plan is to include both of these metrics in our 2016 charity reviews. We expect the “days of suffering” approach to suggest corporate outreach and similar interventions are much more cost-effective than they have seemed in our previous estimates.
We could take this a step farther, and differentiate “days of suffering” between different farming methods, which vary substantially between species. We think that making such conversions would be highly subjective and take a large amount of research to do in a thorough and somewhat reliable way. We hope to do that in the future but aren’t counting on finishing it in time for our 2016 charity reviews.
Adjusting Final Outcomes
Some have suggested that we apply general discount rates to the outputs (e.g. lives spared, days of suffering spared) of our CEEs, such as dividing all the outputs by two. We think these make sense in some contexts, but not others. First, we already discount based on how far into the future an impact will occur. Even if we think an intervention may have policy impacts lasting for many years, we account only for fairly short-term impacts. We elaborate on why we don’t consider long-term impacts in our CEEs in this post, although they are important considerations in the overall evaluation of a charity.
We don’t apply a general discount rate beyond this to our estimates, such as one based on the lack of robust evidence or based on intuitions we have about what the final results should look like. We think a general robustness discount rate would be a reasonable approach, but we aren’t including it for two reasons. First, we already try to account for robustness in the inner workings of our calculations, such as in the estimate of dietary change from veg outreach or in the time period considered for new corporate policies. Second, a general robustness discount would greatly increase the uncertainty in our CEEs and decrease their accuracy, similar to what would happen if we considered long-term impacts.
We’re more strongly opposed to intuition-based discounting, such as reasoning: “The final outcome of this estimate in number of animals spared seems too optimistic to me, so we should either apply a discount rate or reduce the size of some of the inputs.” We don’t expect ourselves, or others, to have reliable intuitions about whether our estimates of “lives spared per dollar” or “days of suffering spared per dollar” are too low or too high. In our everyday lives, we don’t encounter figures of these sorts that could help us build useful intuitions. Therefore, we expect our best estimates to come from adjusting the values of earlier inputs for which we have better intuitions or evidence.
We also see the results of our CEEs as one perspective on the total effectiveness of an intervention rather than a representation of our overall views. If we took the latter approach, it might make sense to adjust final values based on intuition. Instead, we incorporate some of these intuitions into the qualitative part of our review and allow the CEEs to feed unadulterated into our overall views.
Sharing Different Staff Estimates
We believe that taking a variety of perspectives is key to making progress on the difficult questions in animal advocacy. One way to do this for our charity evaluations would be by sharing different estimates of the factors in our CEEs. Given that multiple staff members at ACE have done substantial research into this area and already have developed views on these estimates, it would be relatively easy for us to publish a survey of these perspectives. It would still take a substantial amount of time to set up these estimates, and while we are unsure whether or not we will prioritize doing this in 2016, we hope to do it eventually.
For example, the Open Philanthropy Project notes their skepticism in their recent write-up of a grant to The Humane League: “As a point of comparison, Animal Charity Evaluators (ACE) estimates that its top charities (including the Humane League) spare 13-14 animals an average of about a third of a year of factory farming per dollar spent across their full range of interventions.8 We do not have much confidence in the accuracy of these estimates, but if they were accurate, and if sparing a hen a year of battery cage confinement were equivalent to sparing an animal a third of a year of life on a factory farm altogether, corporate cage free campaigns would be many times more cost-effective.”
Hopefully we’ll have better figures for predicting consumer behavior in the future, through our new Advocacy Research Program and other research efforts. However, the reliability of these figures will likely continue to be limited (for a number of reasons, such as reliance on self-report and limited time horizons) and qualitative analysis will probably still play an important role.
This difference could result from having better evidence available in assigning the potential value of an activity. It could also result from a general effect where large numbers are common in animal advocacy (e.g. the number of animals slaughtered per year, the number of animals who benefit from a new company policy), but small probabilities are less common so assigning them seems more overconfident.
We think the results of our CEEs for our top charities are too close together to be used this way, given our levels of uncertainty on the specific estimates involved. Although minor differences in CEE results are non-zero evidence of a difference in effectiveness, we think they are very weak evidence that can be easily outweighed by qualitative considerations that are not factored into our CEEs.
We recognize that we can have a more adaptable perspective and think of our range in both qualitative and quantitative terms. Indeed, surprise can be thought of as an estimate of % likelihood of obtaining a certain value. If we had to put a probability on how often we expect an estimate to fall outside of our “prediction interval,” we would put this at 10%. We expect this probability framing to be more useful as we gather more data over the years to vet our own estimates, and we expect the surprise framing to be more useful in communication and creating initial estimates.
Filed Under: Research Tagged With: numbers, our thinking
About Jacy Reese
Jacy spends most of his time researching and promoting effective social change. He's been active in the effective altruism community for many years and would love to talk to you about how you can do the most good with your career, donations, or activism.